AI might have already set the stage for the next tech monopoly
23 March, 2023
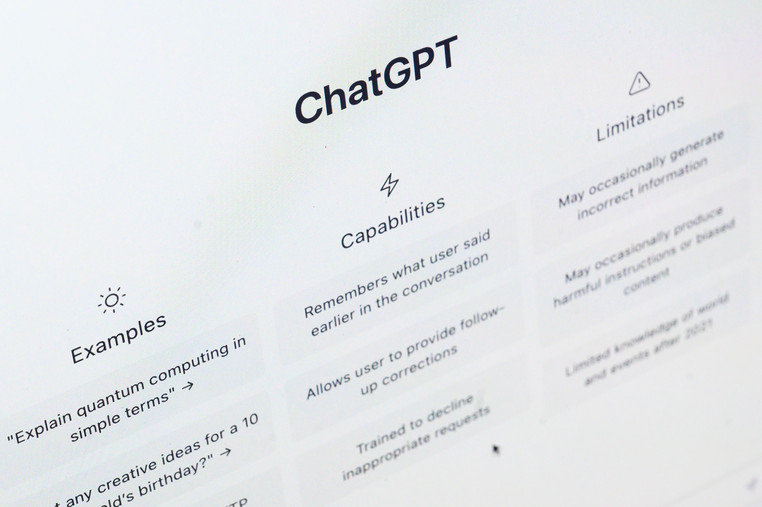
As generative AI and its eerily human chatbots explode into the public realm — including Google’s Bard, released yesterday — Silicon Valley looks ripe for another big era of disruption.
Think about the era of personal computers, or online businesses, or social platforms, when an accessible, unpredictable new idea shakes up the establishment.
But unlike earlier disruptions, the reality of the generative AI race is already looking a little … top-heavy.
With AI, the big innovation isn’t the kind of cheap, accessible technology that helps garage startups grow into world-changing new companies. The models that underpin the AI era can be extremely, extremely expensive to build.
Now some thinkers and policymakers are starting to worry that this could be the first “disruptive” new tech in a long time built and controlled largely by giants — and which could entrench, rather than shake up, the status quo.
This message even got to Congress earlier this month, when MIT artificial intelligence expert Alexandr Madry told a House subcommittee that in the emerging AI ecosystem, a handful of large AI systems were becoming the difficult-to-replicate foundations on top of which other systems are being built. He warned that “very few players will be able to compete, given the highly specialized skills and enormous capital investments the building of such systems requires.” The same week, Rep. Jay Obernolte (R-CA), the only member of Congress with a master’s degree in artificial intelligence, told Politico’s Deep Dive that he worries “about the ways that AI can be used to create economic situations that look very and act very much like monopolies.”
The concern right now is largely about the “upstream” part of AI, where the large generative AI models and platforms are being built. Madry and others are more optimistic about the “almost Cambrian explosion of startups and new use cases downstream of the supply chain,” as Madry put it to Congress.
But that whole ecosystem is dependent on a few big players at the top.
One big reason the AI world is shaping up this way is data: it is bruisingly expensive to train a new AI system from scratch, and only a few companies — primarily the world’s tech giants — have access to enough data to do it well. High-quality data is “the key strategic advantage that they hold, compared to the rest of the world,“ Madry said in an interview with Politico before his Capitol Hill appearance. And when it comes to AI, “better data always wins.”
Madry estimates that it costs hundreds of millions — maybe billions — of dollars to fund the R&D and training for a fully new large language model like GPT-4, or an image generation model like DALL-E 2.
Right now, only a handful of companies — including Google, Meta, Amazon and Microsoft (through their $10 billion investment in OpenAI) — are responsible for the world’s leading large language models, entrenching their upstream advantage in the AI era. In fact, the largest language model reportedly capable of performing better than GPT-3 not developed by a corporation is at a university in Beijing — effectively, a national research project by China.
So, what’s wrong with only having a few players at the top?
For one thing, it creates a much less robust foundation for a major growth area in the tech economy. “Imagine for instance, if one of these large upstream models goes suddenly offline. What happens downstream?” Madry said in the House subcommittee hearing.
For another, big, privately developed models still have problems with biased outputs. And those privately held, black box models are hard to avoid even in academia-led efforts to democratize access to generative AI. Stanford University came out last week with a large language model called Alpaca-LoRA that performs similarly to GPT 3.5 and took only a fraction of the cost to train — but Stanford’s effort is built on top of a pre-trained LLaMA 7B model developed by Meta. The Stanford researchers also noted that they could “likely improve our model performance significantly if we had a better dataset.”
So with “competition” a buzzword in Washington, and leaders newly interested in breaking up monopolies and keeping a lively ecosystem, what can policymakers do about AI? Is there a way to prevent the hottest new technology from simply cementing the power of the tech giants?
One potential government solution would be to create a public resource that allows researchers to understand this technology’s emerging capabilities and limitations. In AI, that would look like building a publicly funded large language model with accompanying datasets and computational resources for researchers to play around with.
There’s even a vehicle for talking about this: The National AI Initiative Act of 2020 appointed a government task force - called the National AI Research Resource — to figure out how to give AI researchers the resources and data they need.
But in deciding how to move forward, the NAIRR Task Force went a different route, choosing to build a “broad, multifaceted, rather diffused platform” over a “public version of GPT,” said Oren Etzioni, one of the members of the task force.
The NAIRR Task Force’s final roadmap, published in late January, recommended that the bulk of NAIRR’s estimated $2.6 billion budget should be appropriated to multiple federal agencies to fund broadly accessible AI resources. Exactly how NAIRR will provide the high-quality training and test data crucial for AI development (especially in building large language models) has been left to a future, independent “Operating Entity” to figure out.
Etzioni disagreed with the task force’s decision, calling NAIRR’s choice to move away from building a public version of a foundation generative model “a huge mistake.” And while he respects the decision to tackle a broad range of AI R&D problems, the issue with the NAIRR roadmap, he said, was “a lack of focus.”
But Etzioni says he doesn’t think it’s “game over” for a more democratized AI competition landscape. Enter the open-source developers — people who build software with publicly accessible source code as a matter of principle. “One should never underestimate the ability of the open source community,” he said, pointing to the large language model BLOOM, an open-access ChatGPT rival developed by VC-backed AI startup Hugging Face.
Hugging Face recently entered a partnership with Amazon Web Services to make their AI tools available to AWS cloud customers.
Source: www.politico.com
TAG(s):